多尺度目标检测
%matplotlib inline
from PIL import Image
import numpy as np
import torch
import sys
sys.path.append("..")
import d2lzh_pytorch as d2l
print(torch.__version__) # 1.2.0
img = Image.open('../../docs/img/catdog.jpg')
w, h = img.size
w, h
1.11.0+cu113
(728, 561)
d2l.set_figsize()
def display_anchors(fmap_w, fmap_h, s):
# 前两维的取值不影响输出结果(原书这里是(1, 10, fmap_w, fmap_h), 我认为错了)
fmap = torch.zeros((1, 10, fmap_h, fmap_w), dtype=torch.float32)
# 平移所有锚框使均匀分布在图片上
offset_x, offset_y = 1.0/fmap_w, 1.0/fmap_h
anchors = d2l.MultiBoxPrior(fmap, sizes=s, ratios=[1, 2, 0.5]) + \
torch.tensor([offset_x/2, offset_y/2, offset_x/2, offset_y/2])
bbox_scale = torch.tensor([[w, h, w, h]], dtype=torch.float32)
d2l.show_bboxes(d2l.plt.imshow(img).axes,
anchors[0] * bbox_scale)
准备皮卡丘数据集
import os
import json
from tqdm import tqdm
import numpy as np
import matplotlib.pyplot as plt
from mxnet.gluon import utils as gutils # pip install mxnet
from mxnet import image
data_dir = '../../data/pikachu'
os.makedirs(data_dir, exist_ok=True)
def _download_pikachu(data_dir):
root_url = ('https://apache-mxnet.s3-accelerate.amazonaws.com/'
'gluon/dataset/pikachu/')
dataset = {'train.rec': 'e6bcb6ffba1ac04ff8a9b1115e650af56ee969c8',
'train.idx': 'dcf7318b2602c06428b9988470c731621716c393',
'val.rec': 'd6c33f799b4d058e82f2cb5bd9a976f69d72d520'}
for k, v in dataset.items():
gutils.download(root_url + k, os.path.join(data_dir, k), sha1_hash=v)
if not os.path.exists(os.path.join(data_dir, "train.rec")):
print("下载原始数据集到%s..." % data_dir)
_download_pikachu(data_dir)
def load_data_pikachu(batch_size, edge_size=256): # edge_size:输出图像的宽和高
train_iter = image.ImageDetIter(
path_imgrec=os.path.join(data_dir, 'train.rec'),
path_imgidx=os.path.join(data_dir, 'train.idx'),
batch_size=batch_size,
data_shape=(3, edge_size, edge_size), # 输出图像的形状
# shuffle=False, # 以随机顺序读取数据集
# rand_crop=1, # 随机裁剪的概率为1
min_object_covered=0.95, max_attempts=200)
val_iter = image.ImageDetIter(
path_imgrec=os.path.join(data_dir, 'val.rec'), batch_size=batch_size,
data_shape=(3, edge_size, edge_size), shuffle=False)
return train_iter, val_iter
batch_size, edge_size = 1, 256
train_iter, val_iter = load_data_pikachu(batch_size, edge_size)
batch = train_iter.next()
batch.data[0][0].shape, batch.label[0][0].shape
((3, 256, 256), (1, 5))
转换成PNG图片并保存
def process(data_iter, save_dir):
"""batch size == 1"""
data_iter.reset() # 从头开始
all_label = dict()
id = 1
os.makedirs(os.path.join(save_dir, 'images'), exist_ok=True)
for sample in tqdm(data_iter):
x = sample.data[0][0].asnumpy().transpose((1,2,0))
plt.imsave(os.path.join(save_dir, 'images', str(id) + '.png'), x / 255.0)
y = sample.label[0][0][0].asnumpy()
label = {}
label["class"] = int(y[0])
label["loc"] = y[1:].tolist()
all_label[str(id) + '.png'] = label.copy()
id += 1
with open(os.path.join(save_dir, 'label.json'), 'w') as f:
json.dump(all_label, f, indent=True)
process(data_iter = train_iter, save_dir = os.path.join(data_dir, "train"))
process(data_iter = val_iter, save_dir = os.path.join(data_dir, "val"))
下载数据集
assert os.path.exists(os.path.join(data_dir, "train"))
读取数据集
# 本类已保存在d2lzh_pytorch包中方便以后使用
class PikachuDetDataset(torch.utils.data.Dataset):
"""皮卡丘检测数据集类"""
def __init__(self, data_dir, part, image_size=(256, 256)):
assert part in ["train", "val"]
self.image_size = image_size
self.image_dir = os.path.join(data_dir, part, "images")
with open(os.path.join(data_dir, part, "label.json")) as f:
self.label = json.load(f)
self.transform = torchvision.transforms.Compose([
# 将 PIL 图片转换成位于[0.0, 1.0]的floatTensor, shape (C x H x W)
torchvision.transforms.ToTensor()])
def __len__(self):
return len(self.label)
def __getitem__(self, index):
image_path = str(index + 1) + ".png"
cls = self.label[image_path]["class"]
label = np.array([cls] + self.label[image_path]["loc"],
dtype="float32")[None, :]
PIL_img = Image.open(os.path.join(self.image_dir, image_path)
).convert('RGB').resize(self.image_size)
img = self.transform(PIL_img)
sample = {
"label": label, # shape: (1, 5) [class, xmin, ymin, xmax, ymax]
"image": img # shape: (3, *image_size)
}
return sample
# 本函数已保存在d2lzh_pytorch包中方便以后使用
def load_data_pikachu(batch_size, edge_size=256, data_dir = '../../data/pikachu'):
"""edge_size:输出图像的宽和高"""
image_size = (edge_size, edge_size)
train_dataset = PikachuDetDataset(data_dir, 'train', image_size)
val_dataset = PikachuDetDataset(data_dir, 'val', image_size)
train_iter = torch.utils.data.DataLoader(train_dataset, batch_size=batch_size,
shuffle=True, num_workers=4)
val_iter = torch.utils.data.DataLoader(val_dataset, batch_size=batch_size,
shuffle=False, num_workers=4)
return train_iter, val_iter
batch_size, edge_size = 32, 256
train_iter, _ = load_data_pikachu(batch_size, edge_size, data_dir)
batch = iter(train_iter).next()
print(batch["image"].shape, batch["label"].shape)
torch.Size([32, 3, 256, 256]) torch.Size([32, 1, 5])
图示数据
imgs = batch["image"][0:10].permute(0,2,3,1)
bboxes = batch["label"][0:10, 0, 1:]
axes = d2l.show_images(imgs, 2, 5).flatten()
for ax, bb in zip(axes, bboxes):
d2l.show_bboxes(ax, [bb*edge_size], colors=['w'])
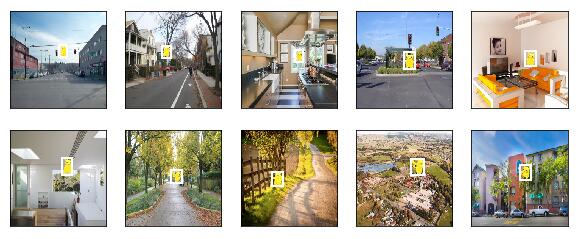
Comments NOTHING