import torch
print(torch.__version__)
0.4.1
1.2 自动求梯度
1.2.1 概念
上一节介绍的Tensor
是这个包的核心类,如果将其属性.requires_grad
设置为True
,它将开始追踪(track)在其上的所有操作。完成计算后,可以调用.backward()
来完成所有梯度计算。此Tensor
的梯度将累积到.grad
属性中。
注意在调用.backward()
时,如果Tensor
是标量,则不需要为backward()
指定任何参数;否则,需要指定一个求导变量。
如果不想要被继续追踪,可以调用.detach()
将其从追踪记录中分离出来,这样就可以防止将来的计算被追踪。此外,还可以用with torch.no_grad()
将不想被追踪的操作代码块包裹起来,这种方法在评估模型的时候很常用,因为在评估模型时,我们并不需要计算可训练参数(requires_grad=True
)的梯度。
Function
是另外一个很重要的类。Tensor
和Function
互相结合就可以构建一个记录有整个计算过程的非循环图。每个Tensor
都有一个.grad_fn
属性,该属性即创建该Tensor
的Function
(除非用户创建的Tensor
s时设置了grad_fn=None
)。
下面通过一些例子来理解这些概念。
1.2.2 Tensor
x = torch.ones(2, 2, requires_grad=True)
print(x)
print(x.grad_fn)
tensor([[1., 1.], [1., 1.]], requires_grad=True) None
y = x + 2
print(y)
print(y.grad_fn)
tensor([[3., 3.], [3., 3.]], grad_fn=<AddBackward>) <AddBackward object at 0x10ed634a8>
注意x是直接创建的,所以它没有grad_fn
, 而y是通过一个加法操作创建的,所以它有一个为<AddBackward>
的grad_fn
。
print(x.is_leaf, y.is_leaf)
True False
z = y * y * 3
out = z.mean()
print(z, out)
tensor([[27., 27.], [27., 27.]], grad_fn=<MulBackward>) tensor(27., grad_fn=<MeanBackward1>)
通过.requires_grad_()
来用in-place的方式改变requires_grad
属性:
a = torch.randn(2, 2) # 缺失情况下默认 requires_grad = False
a = ((a * 3) / (a - 1))
print(a.requires_grad) # False
a.requires_grad_(True)
print(a.requires_grad) # True
b = (a * a).sum()
print(b.grad_fn)
False True <SumBackward0 object at 0x10ed63c50>
1.2.3 梯度
因为out
是一个标量,所以调用backward()
时不需要指定求导变量:
out.backward() # 等价于 out.backward(torch.tensor(1.))
print(x.grad)
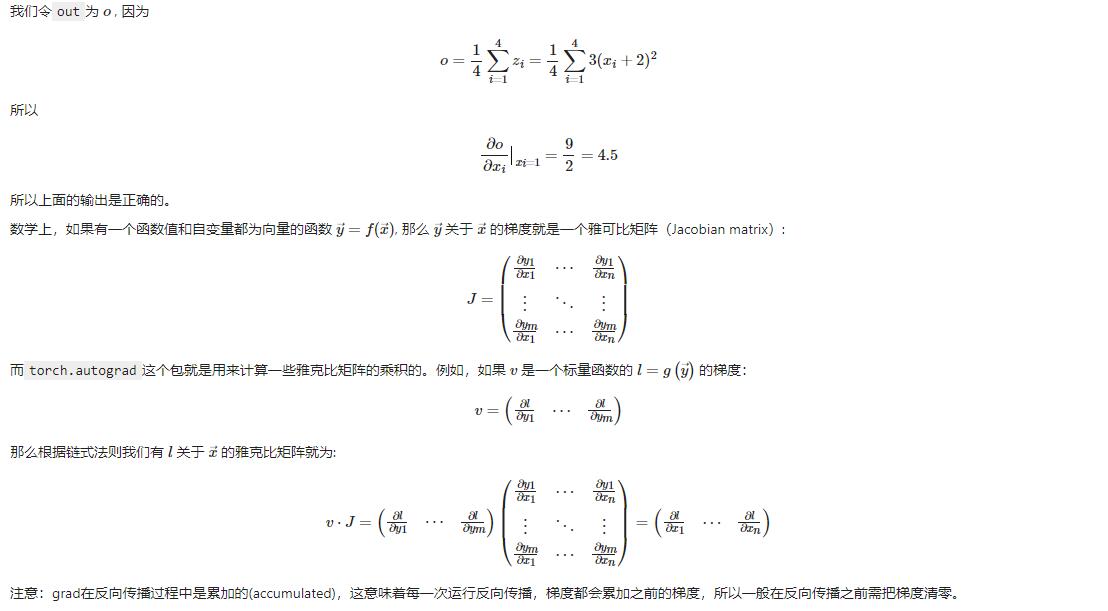
# 再来反向传播一次,注意grad是累加的
out2 = x.sum()
out2.backward()
print(x.grad)
out3 = x.sum()
x.grad.data.zero_()
out3.backward()
print(x.grad)
tensor([[5.5000, 5.5000], [5.5000, 5.5000]]) tensor([[1., 1.], [1., 1.]])
x = torch.tensor([1.0, 2.0, 3.0, 4.0], requires_grad=True)
y = 2 * x
z = y.view(2, 2)
print(z)
tensor([[2., 4.], [6., 8.]], grad_fn=<ViewBackward>)
现在 y
不是一个标量,所以在调用backward
时需要传入一个和y
同形的权重向量进行加权求和得到一个标量。
v = torch.tensor([[1.0, 0.1], [0.01, 0.001]], dtype=torch.float)
z.backward(v)
print(x.grad)
tensor([2.0000, 0.2000, 0.0200, 0.0020])
再来看看中断梯度追踪的例子:
x = torch.tensor(1.0, requires_grad=True)
y1 = x ** 2
with torch.no_grad():
y2 = x ** 3
y3 = y1 + y2
print(x, x.requires_grad)
print(y1, y1.requires_grad)
print(y2, y2.requires_grad)
print(y3, y3.requires_grad)
tensor(1., requires_grad=True) True tensor(1., grad_fn=<PowBackward0>) True tensor(1.) False tensor(2., grad_fn=<ThAddBackward>) True
y3.backward()
print(x.grad)
tensor(2.)
为什么是2呢?y3=y1+y2=x^2+x^3,当 x=1 时 dy3/dx 不应该是5吗?事实上,由于 y2 的定义是被torch.no_grad():
包裹的,所以与 y2 有关的梯度是不会回传的,只有与 y1 有关的梯度才会回传,即 x2 对 x 的梯度。
上面提到,y2.requires_grad=False
,所以不能调用 y2.backward()
。
# y2.backward() # 会报错 RuntimeError: element 0 of tensors does not require grad and does not have a grad_fn
如果我们想要修改tensor
的数值,但是又不希望被autograd
记录(即不会影响反向传播),那么我么可以对tensor.data
进行操作.
x = torch.ones(1,requires_grad=True)
print(x.data) # 还是一个tensor
print(x.data.requires_grad) # 但是已经是独立于计算图之外
y = 2 * x
x.data *= 100 # 只改变了值,不会记录在计算图,所以不会影响梯度传播
y.backward()
print(x) # 更改data的值也会影响tensor的值
print(x.grad)
tensor([1.]) False tensor([100.], requires_grad=True) tensor([2.])
Comments NOTHING